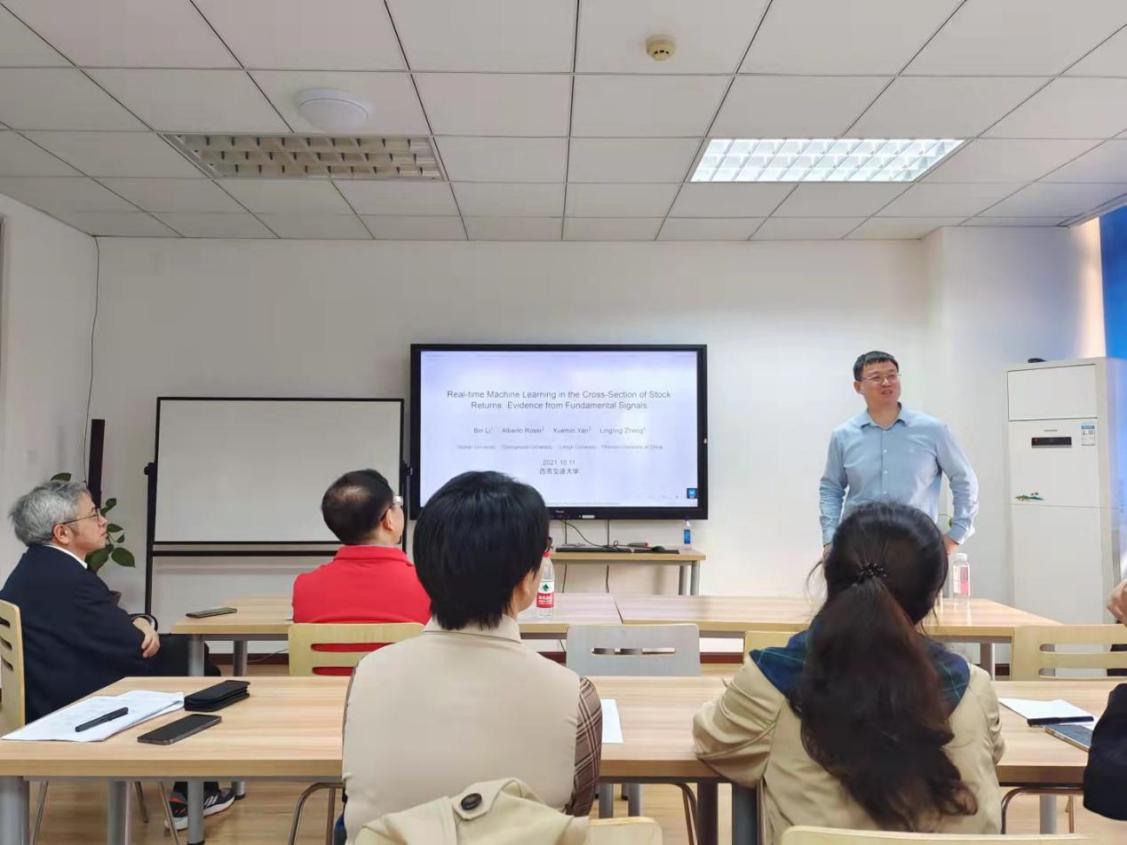
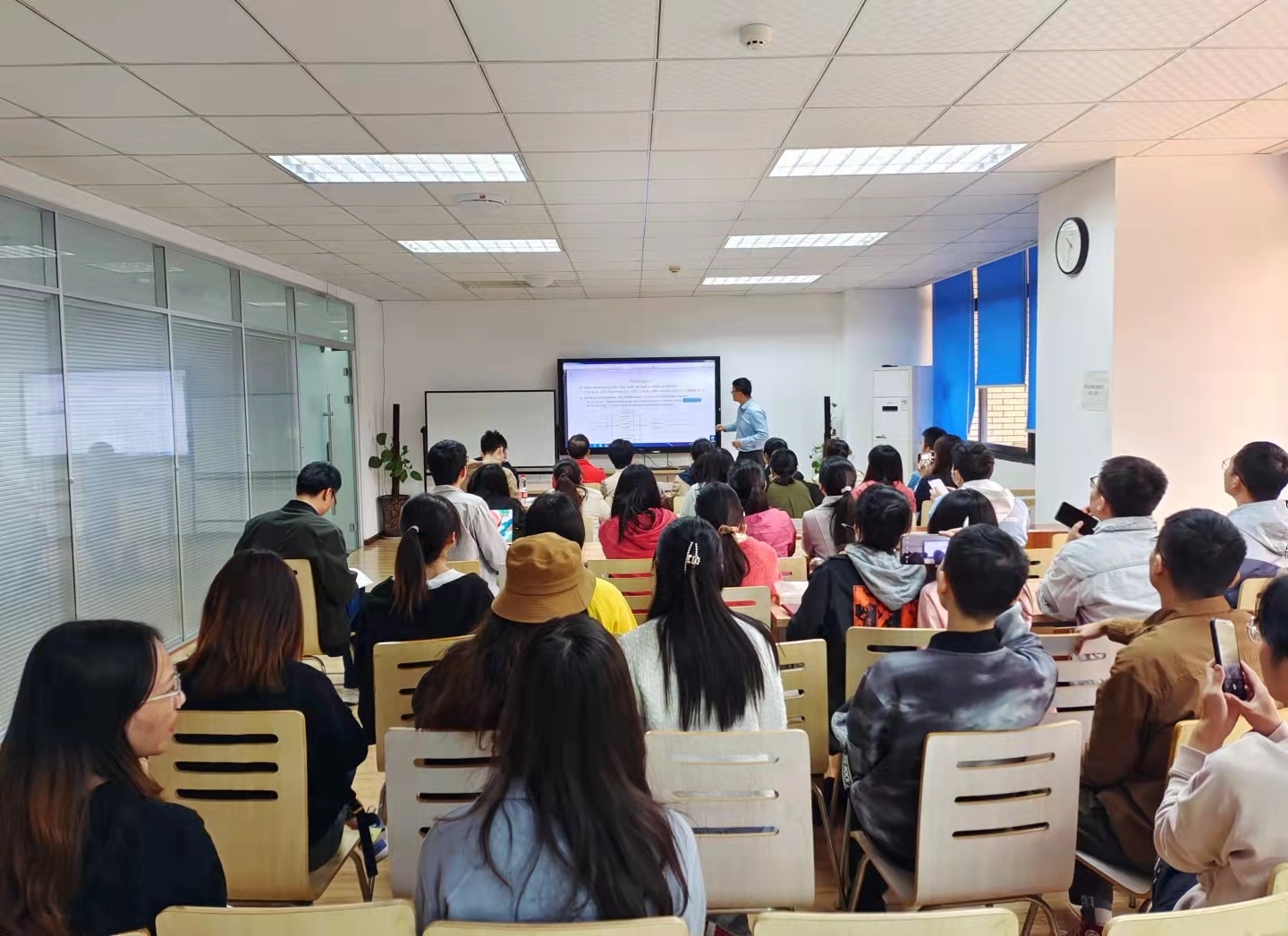
Date: October 11th, 2021
Time: 10:00 am
Venue: Room 0411, Teaching Building 0#, Jiuli Campus
Event Details:
Lecturer: Professor LI Bin, Wuhan University
About the Lecturer:
LI Bin is a professor and doctoral supervisor of the Department of Finance, School of Economics and Management, Wuhan University. He serves as the Deputy Director of the Department of Finance and the Director of the Financial Technology Research Center, the head of the Humanities and Social Sciences Youth Academic Team; a member of the Investment Committee of the Education Development Foundation of Wuhan University and a Chartered Financial Analyst (CFA) licensee. His research interests include financial technology, investment management and machine learning. He has an interdisciplinary background and research capabilities in finance + technology, and he has published papers in financial accounting and artificial intelligence journals and conferences such as: "Journal of Accounting Research", "Artificial Intelligence", "Journal of Machine Learning Research", "Journal of Management Science", "Chinese Industrial Economics", ICML, IJCAI. He presides over one project of National Natural Science Foundation of China and so on. His Foundation Youth Project has been evaluated as excellent after finalization. His research has been reprinted and applied by Columbia University School of Law, Capital Markets.com, Haitong Securities, etc., and was awarded as the most popular article in the economics category of the National People's University of Copying Newspapers and Periodicals, and the third prize for outstanding cooperation projects of the Shanghai Futures Exchange.
About the Lecture:
Recent studies document strong performance of machine learning based investment strategies. These strategies use anomaly variables discovered ex-post as predictors of stock returns and cannot be implemented in real time. We construct machine learning strategies from a “universe” of fundamental signals identified ex-ante and find that their out-of-sample performance is considerably weaker than those documented by previous studies. In addition, we find significant degradation from in-sample performance to out-of-sample performance, supporting the predictions of Martin and Nagel (2020). Overall, our results offer a more tempered view of the practical value of machine learning strategies relative to prior literature.